Entos (Now Iambic) in Collaboration with Caltech and NVIDIA: Advances in geometric deep learning for computational chemistry in Proceedings of the National Academy of Science USA
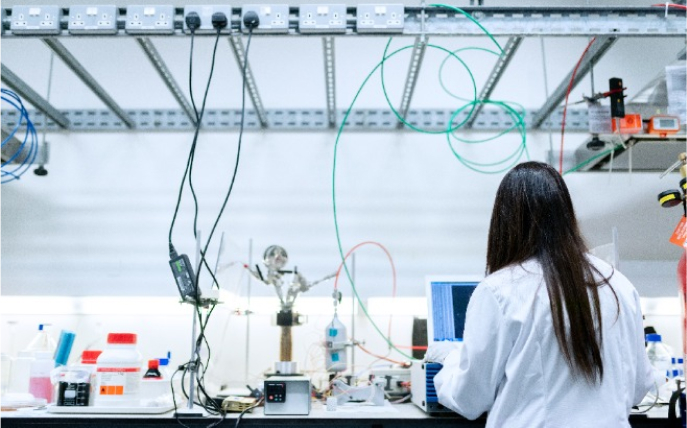
SAN DIEGO, CA – Entos, Inc., the creator of a breakthrough AI-driven high-throughput platform to deliver precision oncology therapeutics announces its latest advances in its OrbNet technology for using geometric deep learning to accurately solve the Schrodinger Equation in the Proceedings of the National Academy of Science USA.
At the heart of the Entos AI-driven HT platform is the proprietary OrbNet technology, which provides unprecedented accuracy across multiple drug-development endpoints with sparse data that can be generated either from computational or experimental sources. The recently announced publication, completed in collaboration with scientists from Entos, Caltech, and NVIDIA, introduces a rigorous framework to conserve the symmetries of quantum chemical systems within deep neural networks. This novel framework greatly improves the prediction of numerous molecular electronic and energetic properties, even for systems far from the training data.
“The Entos AI-driven HT platform leverages the latest advances in physics-informed machine learning to deliver better drug molecules, faster. This work illustrates how Entos continues to innovate at the very forefront of AI-driven drug discovery, and in collaboration with leading scientists at Caltech and NVIDIA.”
- Tom Miller, Ph.D, Entos Co-Founder and Chief Executive Officer
Existing machine learning techniques are challenged by the scarcity of training data when exploring unknown chemical spaces. Entos OrbNet overcomes this barrier by systematically incorporating knowledge of molecular electronic structures into deep learning. By developing a physics-inspired equivariant neural network, the method learns molecular representations based on the electronic interactions among atomic orbitals. With the advances reported in the paper, OrbNet accurately models a wide spectrum of target properties while being several orders of magnitude faster than state-of-the-art quantum mechanical methods based on density functional theory. Despite only using training samples collected from readily available small-molecule libraries, OrbNet outperforms traditional semiempirical and machine learning–based methods on comprehensive downstream benchmarks that encompass diverse main-group chemical processes. OrbNet also describes interactions in challenging charge-transfer complexes and open-shell systems. This work creates new opportunities for physics-informed machine learning in chemistry and drug-discovery, where the acquisition of experimental or reference training data is prohibitively costly.
Full Article: https://www.pnas.org/doi/abs/10.1073/pnas.2205221119
About Entos
Headquartered in San Diego, California, Entos is disrupting drug discovery with its cutting-edge platform that uses AI-driven high-throughput experimentation to rapidly develop small-molecule therapeutics. The Entos team is comprised of renowned scientists and engineers with world-class expertise in machine learning, medicinal chemistry, high-throughput experimentation, and drug discovery. Learn more about Entos's industry-revolutionizing platform at www.entos.ai.
Media Contacts
TJ White
Sard Verbinnen & Co.
Entos-SVC@sardverb.com